Advancing Cybersecurity and AI-Driven Detection Techniques has become a top priority for individuals and enterprises in today’s digital era. Traditional approaches to threat classification and avoidance find it tricky to keep up with the explosive growth of cyber threats. With its imaginative ways to improve cyber security and military protection, artificial intelligence (AI) has become a troublemaking force. The helpfulness, difficulty, and promise to alter the cyber security of AI-driven detection techniques are all examined in this article.
The Increase of Advancing Cyber-threats
Cyber attacks, which are objective corporations, personal data, and vital infrastructures, are increasingly difficult daily. Malevolent actors use a variety of strategies, including ransomware, phishing assaults, malware, and highly developed persistent threats (APTs). A recent study approximated that by 2025, the annual compensation from cybercrime would total $10.5 trillion internationally, underscoring the critical need for physically powerful refuge actions.
Predictable cyber security methods, such as uncovering based on the signature, use stipulated patterns to find threats. These systems work well against known threats but frequently fail when faced with polymorphic malware or zero-day attacks. AI-powered methods are becoming more widely acknowledged as crucial resources for tackling these issues.
An Impression of AI in Advancing Cybersecurity
Because Advancing Cybersecurity AI allows systems to learn, familiarize you, and look forward to threats, cyber security undergoes an essential shift. Neural networks, machine learning (ML), and normal language meting out (NLP) are important aspects of artificial intelligence (AI) in cyber security. These technological advancements enable firms to:
Find odd trends in user behaviour or network traffic to identify abnormalities.
Forecast future dangers: Recognize any weaknesses before they are taken advantage of.
Automate responses: By automating threat mitigation procedures, response times can be decreased.
Advanced detection methods have been developed due to AI integration; these are covered in more depth below.
AI-Powered Detection Methods
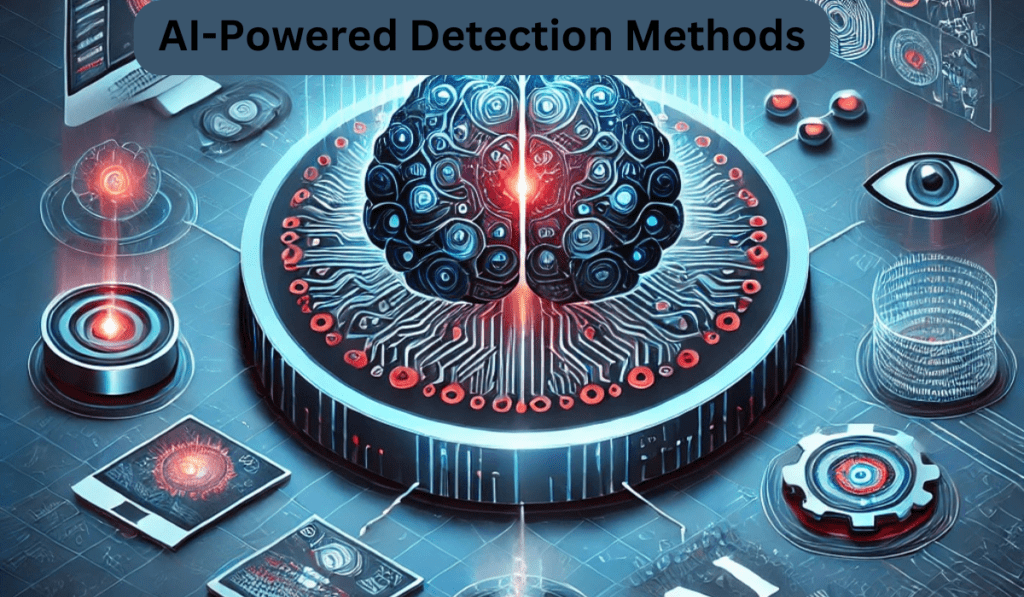
1. Finding Anomalies
One of the most used Advancing Cybersecurity AI methods in cyber security is anomaly detection. It entails spotting departures from accepted standards or patterns pointing to possible dangers. Machine learning algorithms like cluster and unsubstantiated knowledge are normally used.
Use case: interruption discovery systems (IDS) use anomaly discovery to check network transfer and recognize dubious activity.
Benefits: Identifies previously undiscovered threats and adjusts to changing assault methods.
Problems: Excessive false-positive rates may cause alert fatigue, necessitating additional algorithmic improvement.
2. Analytics of Behavior
Analyzing user and object behaviour to find irregularities suggestive of harmful activity is the main goal of behavioural analytics. By utilizing Advancing Cybersecurity AI, systems can identify outliers and provide baseline profiles for typical behaviour.
Use case: Recognizing compromised accounts and insider threats.
Benefits: Reduces false positives by offering context-aware detection.
Challenges: Extensive datasets and ongoing learning are needed to ensure accuracy.
3. NLP, or Natural Language Processing
NLP is crucial in thwarting phishing and social engineering assaults because it allows Advancing Cybersecurity AI systems to comprehend and interpret human language.
Use case: Phishing attempt detection email filtering systems.
Benefits: Increases detection precision by comprehending linguistic patterns.
Challenges: Complex phishing techniques and subtleties in language can occasionally evade detection.
4. Deep Learning
Neural networks are used in deep knowledge and machine learning to process massive volumes of data and find concealed patterns. Real-time monitoring, threat cleverness, and malware detection are several of its uses in cybersecurity.
Use case: Examining file structures and activities to detect polymorphic malware.
Benefits: Excellent precision in identifying intricate dangers.
Difficulties: Needs a lot of training data and is computationally demanding.
Fifth, Threat Hunting Assisted by AI
Proactively looking for possible dangers within an organization’s systems is known as threat hunting. Advancing Cybersecurity AI improves this procedure by automating data processing and ranking alarms according to danger levels.
Use case: Finding hidden malware and advanced persistent threats (APTs).
Benefits: Reduces manual labour and increases detection efficiency.
Challenges: Maintaining efficient threat mitigation requires balancing automation and human skills.
Application of AI in Cybersecurity in the genuine World
Advancing Cybersecurity AI-powered methods are by now having a big bang on several cybersecurity domains:
Monetary Sector: Advancing Cybersecurity AI is used by banks and other financial organizations to secure responsive customer data and recognize fake behaviour.
Healthcare: AI aids in defensive electronic health minutes (EHRs) next to hackers and illegal access.
Critical communications: Advancing Cybersecurity AI is used in water-provide networks, transport systems, and authority grids to monitor intimidation and react to real-time incidents.
Venture Security: The company uses AI to protect thinker property, stop data breaches, and ensure legal compliance.
Obstacle and Boundaries
Despite AI’s huge potential, there are some obstacles to its extensive use in cybersecurity:
Data Privacy: Since Advancing Cybersecurity AI systems need a large amount of data to be taught, data privacy and fulfilment are issues.
Adversarial Attacks: By changing algorithms to avoid uncovering, cybercriminals’ strengths take benefit of weaknesses in AI systems.
Reserve Intensity: Smaller firms may not be capable of having enough money, substantial computational resources, and the particular knowledge necessary to put into practice AI-driven solutions.
False Positives: Since too many bogus positives can excess security workers, complementary compassion and specificity are still a crucial challenge.
AI’s Potential in Cybersecurity
Technological breakthroughs and an increase in cyber threats are predicted to boost the progress of AI in cybersecurity. Imperative trends to keep an eye on are:
Understandable AI (XAI): civilizing accountability and trust by rising directness in AI decision-making.
Federate Learning: Allowing enterprises to train models jointly without exchanging private in sequence.
Using AI to improve Security Orchestration, mechanization, and Response (SOAR) systems for wide-ranging threat administration is known as AI-orchestrated Response.
Creating artificial intelligence (AI) methods to conquer the difficulty that quantum computing presents to conservative encryption is known as “quantum-resistant algorithms.”
Read also: “Digital Detox for Success“
Conclusion
With their unmatched ability to identify, stop, and react to new threats, AI-driven detection approaches are transforming the cybersecurity industry. Even though there are still obstacles, continued study and development should be able to overcome them, guaranteeing AI’s place at the forefront of cybersecurity developments. By integrating AI, organisations may strengthen their defences, safeguard important assets, and maintain an advantage in the always-changing fight against cybercrime.