Regarding monitorability, artificial intelligence is the aptitude to watch, calculate, and analyze AI systems’ shows, presentations, and decision-making processes. Effectual monitoring is more significant than ever as AI technologies spread throughout sectors, including healthcare, banking, and driverless cars. Developers, businesses, and narrow agencies are increasingly concerned about ensuring the monitorability of AI systems in a translucent, safe, and moral method.
This commentary will argue the need for monitorability in AI, the difficulty it poses, and the method for civilizing answerability and intelligibility in AI systems.
In AI, what is monitorability?
In the artificial intelligence framework, monitorability in AI refers to the aptitude to ad infinitum or retrospectively observe and estimate the decisions and behaviors of an AI system. This entails observing an eye on the system’s outputs, figuring out how it made convinced judgments, spotting irregularities or mistakes, and making sure it complies with moral guidelines. By offering insights into how AI systems make decisions and if they act suitably in agreement with the recognized objectives, effectual monitoring guarantees that the AI system’s purpose is as planned.
For example, monitorability is essential in Monitorability in AI-driven healthcare apps to track how AI algorithms help doctors, suggest therapies, or detect medical disorders. The AI system’s decision-making in autonomous cars must also be closely observed to avoid mishaps or malfunctions.
Why is Monitorability Important for AI?
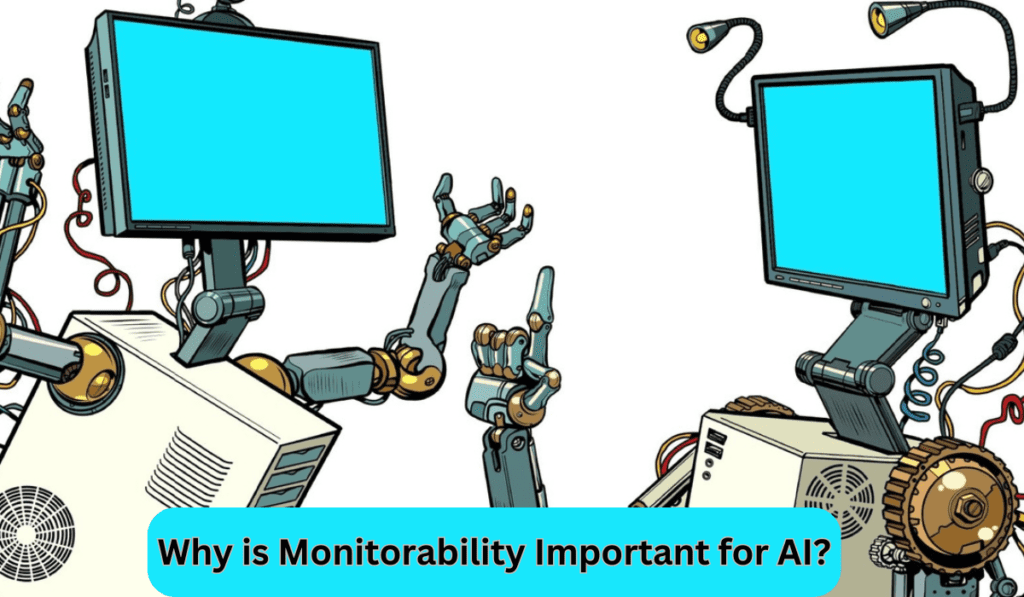
1. Trust and Transparency
Clearness is essential to extensively using and receiving Monitorability in AI systems. The decision-making procedure and rationale of AI systems must be unspoken by users and stakeholders. Monitorability in AI systems may be called “black boxes” with thick and challenging-to-understand decision-making processes if they are not monitorable, eroding trust. AI systems become crystal clear when they are passably monitored, allowing stakeholders to evaluate the logic following these systems’ decisions.
2. Ethical and Accountability Issues
AI choices have real-world repercussions, chiefly in high-stakes industries like healthcare or money. A monitoring system is necessary for formative analysis of what goes wrong if an AI system makes a bad or mistaken choice. This is essential for answerability because it enables developers to track decision-making procedures and correct ethical issues or errors. It would be taxing to hold Monitorability in AI systems responsible for their acts without efficient monitoring, which raises questions regarding justice and fairness.
3. Dependability and Safety
AI systems occasionally show random behavior, chiefly when presented with work of fiction data or circumstances. With incessant monitoring, developers can identify and fix any irregularity or malfunction in real time, guaranteeing the system’s dependability and security. For instance, continuousMonitorability in AI system monitoring is essential in self-driving cars to ensure the system responds properly to novel or dangerous road conditions, decreasing the likelihood of collision.
4. Adherence to Regulations
Government and narrow agencies are paying more attention to ensuring AI systems stick to moral and legal standards as the technology becomes more general. For example, the European Union has implemented AI legislation to guarantee that AI systems are crystal clear and safe and uphold essential rights. Organizations can prevent legal evils and fines by obeying this policy through effective monitorability.
5. Enhancement of Performance
Developers can assess the monitorability of AI systems’ performance over time and pinpoint areas for development by keeping a close eye on them. Organizations can improve the system’s accuracy, efficiency, and decision-making capability by monitoring its outputs and identifying trends of inefficiency or inaccuracy. This is crucial in machine learning models, where feedback loops can be utilized to improve predictions and optimize the system.
Challenges in Ensuring Monitorability of AI
1. The intricacy of AI models
Deep learning systems, in particular, can be quite complicated. Monitorability in AI models. Effective monitoring becomes more difficult with increasingly complex models. It can be challenging for humans to completely comprehend how a decision is made because AI systems frequently process enormous volumes of data over multiple layers of neural networks. This complexity makes monitoring difficult because it takes sophisticated tools and methods to follow the model’s underlying operations and behavior.
2. Security and Privacy of Data
It is frequently necessary to access the data that Monitorability in AI systems is processing to monitor them. Sensitive information, including private or confidential company data, might need to be shielded from unwanted access. It can be difficult to balance the security, privacy, and surveillance requirements. Solutions must guarantee that monitoring systems do not breach data privacy regulations or reveal private information to unapproved parties.
3. The ability to scale
It gets harder to oversee Monitorability in AI systems as they are implemented at scale. For instance, AI systems on social media platforms or big businesses must be continuously checked for compliance, fairness, and performance over thousands or millions of interactions. One major challenge is creating scalable monitoring technologies to manage such enormous data quantities.
4. Fairness and Bias
Biases in the data that AI systems are educated on can be reinforced or even made worse. Effective monitoring is necessary to identify these biases and guarantee that AI systems function equitably. However, it is not always easy to spot and fix bias in AI models, particularly when those models are complicated and have difficult-to-understand decision-making processes. Maintaining equity necessitates ongoing system modifications and monitoring, which can be resource-intensive.
5. Monitoring in Real Time
Real-time monitoring is necessary for many AI applications, including healthcare diagnostics and driverless cars. Real-time AI monitoring, though, has its own set of difficulties, such as the obligation for fast data processing, minimal latency, and the ability to react swiftly to discovery. It takes higher monitor tools and communications with real-time input to ensure the system’s operations align with the intended objectives.
Strategies to Improve Monitorability in AI
1. AI that can be explained (XAI)
The objective of understandable AI (XAI), a bough of AI research, is to build AI systems that are easier for people to understand and more see-through. Developers can produce systems that offer understandable justifications for their choices by considering explainability while creating AI models.
This can involve creating natural language explanations, visualizing decision routes, and simplifying models. XAI might greatly increase their monitorability by simplifying monitoring AI systems’ behavior and helping them comprehend how they arrive at particular conclusions.
2. AI Testing and Auditing
AI auditing is methodically assessing AI models to ensure they perform as intended. Frequent testing and auditing can assist in locating any problems like skewed judgment, security flaws, or performance mistakes. During an audit, the data quality may be evaluated, model outputs may be examined, and the AI’s decision-making process may be verified against predetermined standards. Organizations may ensure AI technologies function dependably and ethically by conducting routine audits.
3. Constant Feedback Loops
Unremitting learning is valuable to AI systems, mainly machine education models. By adding fresh data and modifying obtainable models, AI systems can improve over time through continuous feedback loops. Developers may monitor performance and identify problems early using these feedback loops. Feedback loops enhance model performance, monitor the system’s behavior, and ensure it continues to align with human values and objectives.
Read also: What Is Artificial Intelligence and the Memory Wall?
4. Open Data Procedures
Another crucial component of monitorability is ensuring the data used to train AI models is clear and well-documented. Developers can find any sources of bias or mistakes in the system by keeping thorough records of the data collection, processing, and usage processes. Better monitoring is also made possible by using transparent data policies, which enable developers to track problems back to the data’s source.
Conclusion:
Monitorability ensures AI systems are transparent, accountable, and aligned with human values. It enables real-time oversight, detects biases, and fosters trust, making AI reliable and ethical. As AI grows in complexity, embedding robust monitoring mechanisms is crucial to creating systems that benefit society while upholding fairness and accountability.