Analytical chemistry is no exception to how artificial intelligence (AI) revolutionises several scientific fields. Researchers and experts in analytical chemistry are solving complicated issues, increasing precision, and boosting the effectiveness of chemical tests by utilising machine learning (ML), deep learning (DL), and other AI technologies. This article examines artificial intelligence’s present function in analytical chemistry and its developments, difficulties, and possible future directions.
What is Artificial Intelligence in Analytical Chemistry?
Using computational algorithms and models to automate, optimise, and improve the process of chemical analysis is known as artificial intelligence (AI) in analytical chemistry. Analytical chemistry involves identifying and quantifying chemical substances and frequently manages large datasets, sophisticated instruments, and complex experimental setups. AI helps chemists to identify patterns, extract meaningful insights, and predict outcomes that are not immediately apparent through traditional methods.
The following are the main domains in which analytical chemistry uses AI:
Spectroscopic Data Analysis: To determine molecular structures and forecast attributes, AI models may analyse complex spectroscopic data, including Raman, IR, and NMR spectra.
Chromatographic Optimization: To save time and money, algorithms can optimise the separation conditions in GC and HPLC.
Predictive modelling: AI helps forecast stability, toxicity, and chemical interactions, which helps guide experimental design.
Automation: From sample preparation to data interpretation, repetitive jobs are made easier by robotics and AI-powered software.
Developments in AI Uses
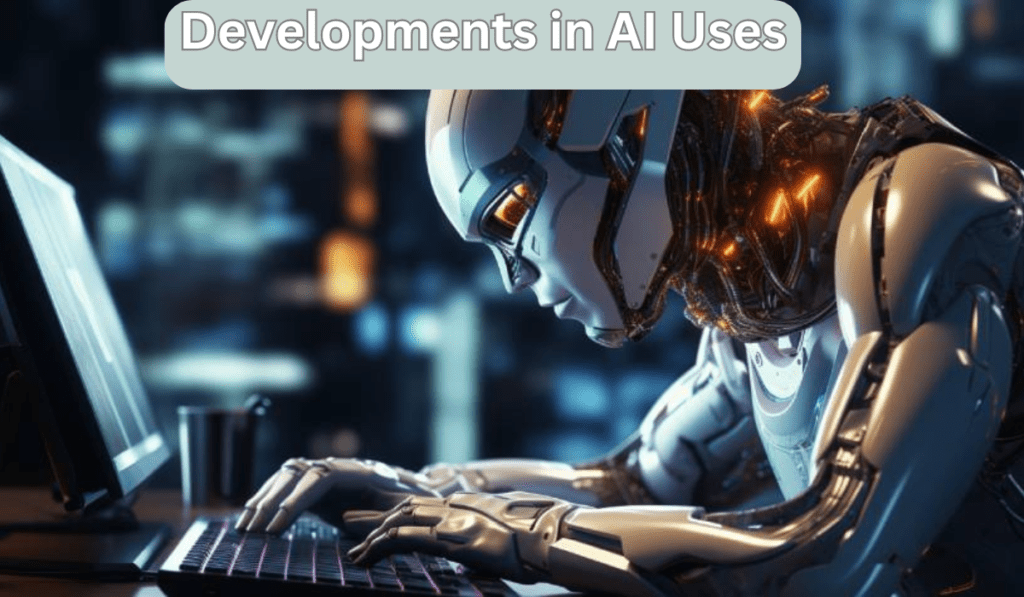
- Artificial intelligence has wholly changed analytical chemistry by developing techniques beyond conventional approaches to speed, accuracy, and adaptability. Some of the most notable developments are listed below:
- Combining Spectroscopy and Machine Learning Spectroscopic data interpretation has benefited dramatically from machine learning methods, especially neural networks. They can detect anomalies, identify unknown substances, and classify complicated datasets. For example, convolutional neural networks (CNNs) are being used to decode complex NMR spectra, significantly reducing the time required for manual processing.
- Integration of Big Data High-dimensional data is frequently dealt with in analytical chemistry. AI models easily handle such data, providing chemists with tools to visualise, evaluate, and interpret results. Chemometrics and AI, for instance, have improved pattern identification and clustering in datasets from high-throughput studies.
- Elevated-Throughput Testing AI streamlines high-throughput experimental operations, allowing for the quick screening of thousands of compounds. Algorithms significantly lessen the experimental burden by recommending the next optimal set of settings based on learning from earlier experiments.
- Instrumentation Driven by AI capabilities is increasingly incorporated in modern equipment. AI-enabled mass spectrometers, for example, may automatically identify and measure target molecules, giving researchers real-time feedback to help them make decisions during studies.
- Environment and Medicine AI applications have advanced environmental monitoring, detecting contaminants and monitoring chemical composition changes. Artificial intelligence (AI) in the pharmaceutical industry speeds up medication research and quality control by forecasting molecular efficacy and detecting production imperfections.
Challenges in Implementing AI
Despite its benefits, integrating AI into analytical chemistry poses several challenges:
Availability and Quality of Data For AI models to be trained effectively, high-quality, carefully selected datasets are necessary. Obtaining such datasets in analytical chemistry can be challenging because of differences in sample types, instrument performance, and experimental circumstances. Unreliable models are the result of poor data quality.
- AI Model Interpretability Many AI methods, such as intense learning, are regarded as “black boxes” because of how opaque their decision-making is. Chemists must comprehend how an AI model makes its decisions, especially in situations involving quality control and regulations.
- Connecting with Current Workflows AI adoption necessitates incorporating it into workflows and established laboratory techniques. This may not always be possible because it may require significant training, software, and hardware adjustments.
- The gap in Skills Both computational science and analytical chemistry expertise are necessary for the efficient use of AI. Many organisations and sectors still struggle to close this transdisciplinary divide.
Ethical and Legal Issues: To guarantee moral AI applications in chemistry, data privacy, algorithmic bias, and intellectual property rights must be resolved.
Prospects for the Future
- With more than a few critical trends and advancements anticipated to influence the regulation, the future of AI in investigative chemistry appears bright:
- Explainable AI (XAI) aims to create translucent and interpretable AI models. Explainable AI will amplify computerised systems’ credibility and use in vital fields like ecological monitoring and pharmaceutical construction.
- Hybrid models: There is a growing trend of combining AI algorithms with conventional chemistry expertise. Hybrid models combine AI-driven predictions with domain-specific rules to improve accuracy and dependability.
- Collaborative Efforts and Open-Source Platforms Initiatives such as collaborative data-sharing platforms and open-source software will democratise access to AI tools, encouraging innovation in the analytical chemistry community.
- Integration with the Internet of Things (Iota) AI-powered Iota devices will enable real-time monitoring and analysis in remote or hazardous environments; for instance, AI-enabled smart sensors could transform on-site environmental testing.
- Intelligent Green Chemistry By streamlining chemical processes to reduce waste and energy consumption, artificial intelligence (AI) can support sustainable practices and integrate analytical chemistry with international environmental goals.
- Training and Education Computational training will be incorporated into educational programs as AI increasingly integrates into analytical chemistry. Workshops, certifications, and multidisciplinary courses will equip the chemists of tomorrow to use AI efficiently.
Benefits of Implementing AI
- There are several advantages to using AI in analytical chemistry that are revolutionising the discipline:
- Increased Productivity AI drastically cuts down on time and labour needs by speeding up procedures like data analysis, equipment calibration, and sample preparation.
- Enhanced Precision and Accuracy AI guarantees more consistent and trustworthy outcomes in chemical studies by reducing human error and automating data interpretation.
- Managing Complicated Datasets Large, multidimensional datasets are easily processed by AI, which reveals patterns and insights that are frequently impossible or difficult to identify with conventional techniques.
- Cutting Expenses Because AI streamlines processes and eliminates experimental repetition, it is a financially appealing choice for labs.
- Artificial Intelligence (AI)-powered solutions facilitate real-time experiment and process monitoring, offering prompt feedback and actionable insights.
- New Approaches to Experimental Design Researchers can concentrate on the most promising directions by using machine learning algorithms and predictive modelling to guide the design of trials.
- Resource Optimization and Sustainability AI promotes greener practices by optimising chemical processes and lowering waste and energy usage
Read also: What Is Artificial Intelligence and the Memory Wall?
Conclusion:
Analytical chemistry is changing due to artificial intelligence’s increased accuracy, efficiency, and capacity to manage extensive, complicated information. Even though there are still issues with data quality, interpretability, and skill gaps, the progress made so far points to a promising future.
Integrating AI into analytical chemistry can open up new opportunities and promote innovation and sustainability in the industry by tackling these issues and embracing emerging trends. AI has the potential to completely transform our understanding of and interactions with the chemical world as its symbiosis with analytical chemistry grows.